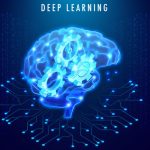
Integrated Sensing and Communication (ISAC) systems provide considerable benefits over traditional communication and sensing networks. However, their design and implementation pose significant challenges, especially in terms of signal processing and computational demands. Combining these functionalities necessitates solving complex optimization problems to create optimal waveforms and process signals for both communication and sensing. These tasks are further complicated by constraints such as limited computational resources, tight timing requirements, and rapidly changing channel conditions. Traditional signal processing methods often fall short in achieving the desired outcomes under these circumstances.
Given the complexity of ISAC systems, alternative strategies are essential, as conventional mathematical and iterative optimization approaches may not suffice. Machine learning (ML), particularly Deep Learning (DL), emerges as a promising solution to address these challenges. By leveraging DL techniques, ISAC systems can reduce computational complexity while achieving near-optimal performance.
The intersection of deep learning and ISAC may provide substantial opportunities to enable the implementation of ISAC systems in future communication networks and reduce their energy consumption.
Our recent preprint on this topic:
Deep Learning-based Techniques for Integrated Sensing and Communication Systems: State-of-the-Art, Challenges, and Opportunities
This article reviews recent studies on deep learning-based (DL-based) techniques for integrated sensing and communication (ISAC) systems. Both sensing and communication functions are necessary for many cutting-edge systems, such as robotics, autonomous driving, and unmanned air vehicles (UAVs). Designing a single platform providing both sensing and communication functions will reduce hardware usage and spectrum congestion while improving the system’s energy efficiency. Combining both functions on the same hardware may result in some challenges that can be efficiently solved via DL-based techniques within limited hardware resources. DL-based techniques can effectively produce near-optimum solutions for waveform design, channel estimation, processing sensing data, data demodulation, eliminating interference, and many other challenges encountered in ISAC systems. Because of the advantages of deep learning, recent studies have focused on developing DL-based techniques in ISAC systems. This article aims to explore the state-of-the-art advancements in this area. It reviews the state-of-the-art DL-based techniques developed to overcome the challenges encountered in ISAC system design and development. Moreover, it presents open research problems and a list of future research topics.