In our previous post, we talked about what is Machine Learning and we said that it is very important and useful in dealing with the massive amount of data. This massive amount of data is known as “Big Data”. However, in order to understand what Big Data is, we must know what is Data.
What is Data?
Data is the quantities, characters, or symbols on which operations are performed by a computer are stored and recorded on magnetic, optical, or mechanical recording media, and transmitted in the form of digital electrical signals[1]. Different types of data can be generated from various sources. For example, we can obtain financial data from financial markets, financial reports, etc. The following figure shows various types of data which can be visualized through a computer device:
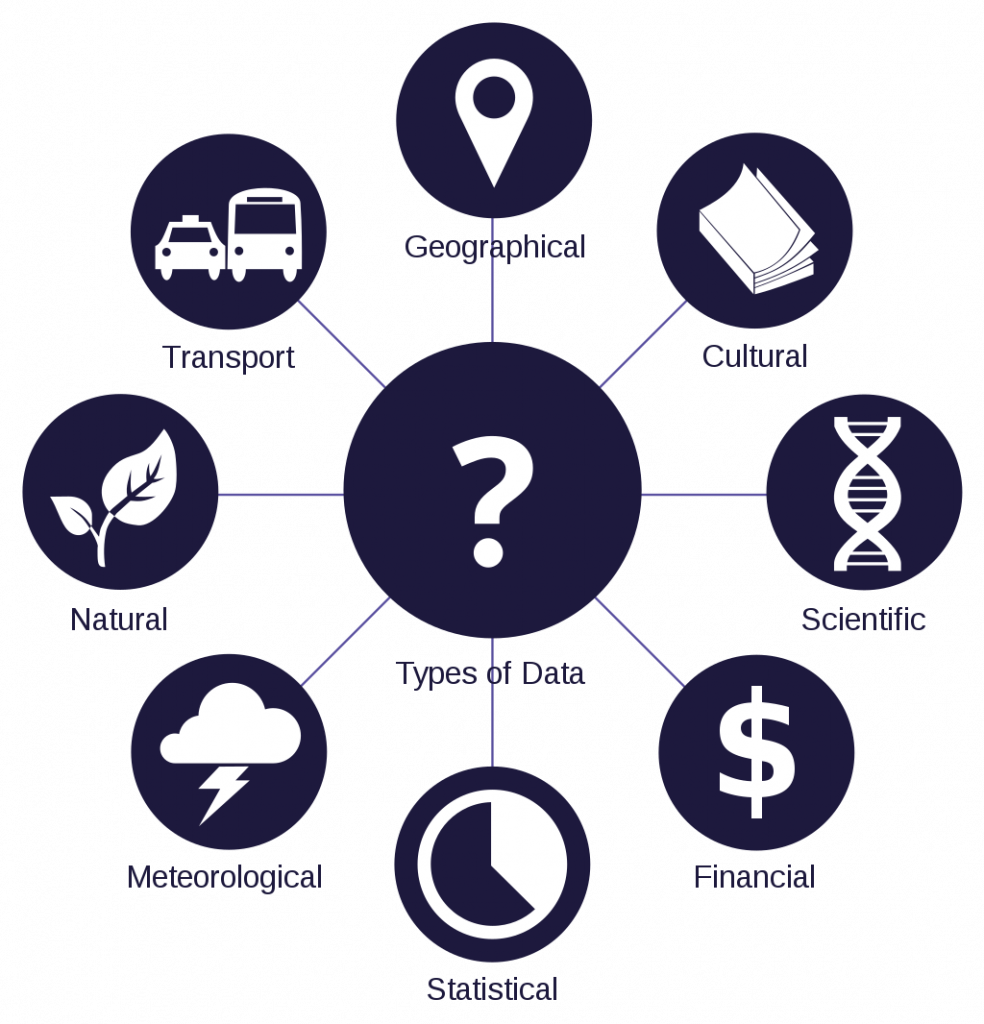
What is Big Data?
According to Gartner, which is the world’s leading information technology research and advisory company, big data is defined as the data that contains greater variety arriving in increasing volumes and with ever-higher velocity. Variety, volumes and velocity is also known as the three V’s. The format of the data could be structured, semi-structured or unstructured based on the source of the data. Let’s give some real life examples.
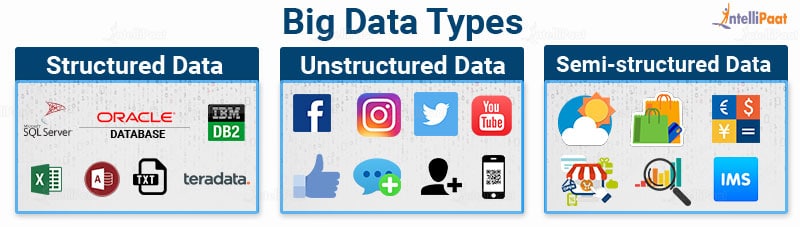
- Approximately, 40 exabytes of data gets generated every month by a single smartphone user. The format of the data is including – but not limited to – texts, phone calls, photos and music.
- In 30 minutes of flight time, a single jet engine can generate more than 10 terabytes of the data. With many thousands of flights per day, the amount of generated data reaches as many as Petabytes.
- The New York Stock Exchange generates about one terabyte of new trade data per day.
The Importance of Big Data
Businesses use big data for all manners of analysis. They collect data in their systems and later used this accumulated data to improve operations, provide better services and increase profitability. They can also obtain valuable insights about their customers as they have more information stored in their systems.
Use Cases
- Predictive Maintenance: By analyzing and predicting the indicators of mechanical failures, such as year and the model of the equipment, the companies can deploy maintenance more cost effectively. Additionally, they can maximize the uptime of the equipments.
- Machine Learning: The availability of the big data offers very important opportunities for Machine Learning algorithms to train their models.
- Comparative Analysis: By examining the user behavior metrics and observing real-time customer engagement, companies can compare their products and services with their competitors.